enture capital investors have always been interested in information technology. Innovations in hardware and software are the foundational sectors on which the venture capital industry was built. However, there is a sector on the horizon that is of increasing interest in the venture space: artificial intelligence. According to IBM, knowledge is doubling every 13 months and artificial intelligence is emerging as a tool to manage the explosion in the volume and variety of data. The trend towards increased venture capital investment in AI is unmistakable and global.

Since 2012, across the OECD, the share of total venture capital invested in AI has risen from under 5% to more than 20% in 2020. Likewise, the value of VC investments in AI firms has also grown considerably:
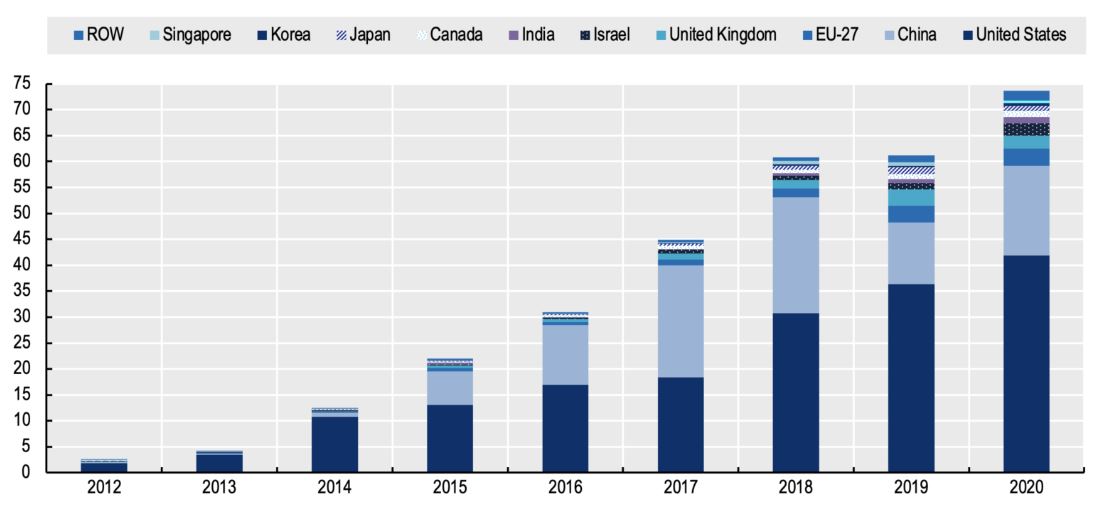
WHAT IS ARTIFICIAL INTELLIGENCE?
Artificial intelligence is the simulation of human intelligence by machines. Some applications include natural language processing, computer vision, speech recognition and analytical capabilities such as knowledge synthesis, inference, and advanced reasoning.
One important subset of artificial intelligence is artificial intelligence for IT Operations aka AIOps. This term was devised by Gartner in 2016 as an industry category for machine learning analytics technology for the enhancement of IT operations analytics. AIOps platforms have two aspects: machine learning and Big Data. To collect the observational data and engagement data that can be found inside a Big Data platform requires a shift away from siloed, segregated IT data models towards a more holistic machine learning-oriented and analytics strategy focused on distilling and implementing the combined IT data.
THE CRISIS IN HEALTHCARE RESEARCH AND DEVELOPMENT
Artificial Intelligence can be a powerful tool across so many different industries but it is particularly useful in managing healthcare data. While knowledge is doubling every 13 months, it is growing the most rapidly in healthcare. According to a 2011 study in Transactions of the American Clinical and Climatological Association, medical knowledge is doubling every 73 days as of 2020. 30% of the global volume of data is generated by the healthcare industry.
At the same time, technological advancements in cloud computing and the Internet of Things have made it much easier to capture and store enormous amounts of patient and medical data digitally. This has resulted in enormous reservoirs of healthcare data—genomic data, healthcare records, medical images, and other patient information—that are an underexploited resource in the healthcare research and development arena. There is a vital need to manage this data and transform it into actionable knowledge to improve the drug discovery process.
A major reason that AI is becoming particularly useful in drug discovery is that there is a growing crisis in the industry. Drugs are notoriously costly resources to develop. An analysis of drug development costs for 98 companies over a 10-year period indicated that the average cost per drug developed and approved by a single drug company was $350 million.
However, for companies that approved between 8 and 13 drugs over 10 years, the cost per drug rose to as much as $5.5 billion. There is mounting evidence that AI can be a powerful tool in mitigating these costs. AI algorithms and machine learning tools can more rapidly, and cost effectively identify new drug applications by deriving insights from cloud-based datasets that are too complex for human interpretation. They can also identify new uses for existing drugs which is inherently appealing to Big Pharma since it is less costly. There is a growing demand for AI applications to help healthcare industry stakeholders understand how to use AI to facilitate faster, more cost-effective drug discovery.
COMMERCIAL IMPACT OF THE AI FIRST MODEL
The results of those firms that have embraced an AI-first model in drug discovery are promising and venture capitalists have taken notice. Already, AI-first biotech companies have more than 150 drugs in discovery and more than 15 in clinical trials with pipelines that have been expanding at an annual rate of approximately 40%. The venture capital deal flow has been impressive with recent financings of companies applying AI in drug discovery including:
- Drug discovery software company Schrodinger received $232 million in a February 2020 IPO backed by Bill Gates and David Shaw.
- AbCellera raised $105 million in a May 2020 Series B funding round to expand its antibody drug discovery platform.
- Relay Therapeutics closed a $400 million IPO in July 2020 to focus on understanding protein motion to design drug candidates.
- Atomwise raised $123 million in Series B funding in August 2020 to support the development of its molecule identification software.
Partnerships are burgeoning across the industry as well:
- In April 2019, AstraZeneca partnered with BenevolentAI to identify new drug candidates for chronic kidney disease and idiopathic pulmonary fibrosis.
- In July 2019, Exscientia brought its AI drug discovery platform Centaur Chemist to a partnership with Rallybio to discover and develop drugs for rare diseases.
- In October 2019, Novartis announced a strategic alliance with Microsoft to apply the latter’s AI algorithms to the former’s large datasets to identify and develop therapeutics.
- In September 2020, Recursion Pharmaceuticals and Bayer announced a drug development partnership to treat fibrotic diseases using Recursion’s AI-guided drug discovery platform.
- in March 2021, Iktos agreed to apply its AI-driven de novo design software to a number of Pfizer’s drug discovery and development programs.
These AI-focused financing and partnership deals are poised to transform and catalyze drug discovery.
WHAT INVESTORS SHOULD CONSIDER: EFFECTIVE IMPLEMENTATION OF AI IN DRUG DISCOVERY AND DEVELOPMENT
It cam be useful to explore how industry stakeholders can effectively implement this powerful tool as that proves a good indication of what investors should consider. Firstly, a transformative AI vision and strategy must be articulated. This will require the participation of senior leadership in developing an overarching leadership vision for AI across the myriad functions of the enterprise, especially discovery and development teams.
Start small and grow gradually while maintaining coordination with the company’s R&D strategy and financial goals. Secondly, a focus on quality data and good technology is required. A trustworthy AI requires high quality data, algorithms, and a strong technology stack for AI use cases. This in turn necessitates a constant organizational commitment to the cleansing, aggregation, and normalization of data across both internal and external sources, as well as the implementation of appropriate data governance and management with a focus on reuse.
However, initially a proof-of-concept algorithm can be developed to simply provide the minimum sufficient analysis to confirm the organization’s ability to extract salient insights from the data in a specific scientific context. After that the lessons learned can be honed and refined to scale and improve the AI technology stack.
Thirdly, external partnerships are critical due to the importance of collaboration in this field. The identification of new AI-native partners and the management of existing partnership can help to accelerate the integration of AI into drug discovery and development processes. This is especially important because in this rapidly changing technology landscape, the amount of financial and human capital as well as new data that is being applied in AI drug discovery is far greater than even the largest of companies can successfully harness on their own.
But pharmaceutical companies will have to adapt to this new reality as they must compete to make themselves desirable partners for AI-native companies which will require making it possible to share data quickly and securely with external actors; fostering a culture of openness and scientific collaboration; and telescoping the time and hassle required to launch partnerships. Fourthly, human capital management increases in importance.
The recruitment, development and retention of AI skill sets to apply to internal projects is essential to maintain competitiveness. This means that human capital management may be too important for the traditional human resources department. Senior leadership must play an active role in defining cross-functional roles that bridge scientific, technical, and business skill sets while also making the workplace attractive to those workers.
Finally, organizational culture is a critical factor in the effective implementation of AI. Due to the speed of change in this sector, a faster pace of work is required with fewer and shorter cycles of drug identification and lead optimization. This means that companies will likely run many more discovery programs simultaneously which necessitates a cultural and operational shift. For example, companies may need to increase the frequency of their portfolio board reviews to accommodate the increased speed of an AI-driven workflow. At the same time, a balance must be struck between the adjustment of existing processes to drive decisions off of AI insights while maintaining required regulatory checks.
All of this requires strong organizational commitment throughout the enterprise to make what can be radical cultural changes and to keep iterating until a successful model is developed. A good venture capital investor understands these factors and looks for them as part of a thorough due diligence process.
SUCCESS STORIES
In spite of the challenges inherent in integrating AI into the drug discovery process, some companies are succeeding in developing novel solutions to important problems. With about $85 million in venture capital financing from the likes of Inovia Capital and TCV, Benschsci is an AI-assisted selection platform that extracts usage evidence from scientific papers and organizes it around antibodies. This is relevant to drug discovery because it addresses the problem of the 50% of research antibodies that simply don’t work in experiments. By using data sources along with biologist-trained machine learning models and bioinformatics ontologies, the Benchschi platform enables scientists to select the right antibodies for their experiments faster which facilitates more efficient drug discovery.
Another AI-native company of note in the drug discovery arena is InstaDeep which has raised over $113 million in funding from companies such as CDIB Capital and Chimera Investment to provide a number of AI-enabled solutions ranging from optimized pattern recognition and GPU-accelerated insights to self-learning decision-making systems. The company’s partners include BioNTech (a developer of personalized immunotherapies to help with the treatment of people with cancer and other devastating diseases), and Seed Group (a Chinese agricultural company that specializes in the breeding, production and promotion of crop seeds such as rice corn, wheat and the like).
InstaDeep’s ability to cultivate a partnership with Seed Group indicates how AI-native firms active in healthcare R&D, which requires competence in both biology and chemistry, can have unexpected, non-linear applications. Finally, Seismic Therapeutics is a biotech firm that is integrating AI across the entire biologics discovery process to accelerate immunology drug development. The company has raised over $100 million from Lightspeed Venture Partners and GV which is a testament to investor confidence in the company. Aside from their focus on AI and drug discovery, what distinguishes all three of these companies is that in the first quarter of 2022, they received substantial investments from Google, which is the tech giant that is the most singularly focused on the healthcare market.
GOOGLE TARGETS HEALTHCARE
Corporate venture capitalists have taken an active interest in the AI market particularly as it relates to the huge healthcare market. In particular, Google has identified artificial intelligence as a strategically important technology that will be a fundamental game-changer in the years ahead. It also happens to be a technology that the company has deep domain expertise in since it informs so much of the core functionality of Google’s search engine. Just as importantly, the healthcare market is sufficiently large enough that success in this space can meet the company’s ongoing need for massive long-term revenue streams.
It should therefore be no surprise that Google is the leading non-healthcare tech giant in AI-enabled healthcare. In November 2021, Alphabet (Google's parent company) launched Isomorphic Labs to leverage artificial intelligence for drug discovery. Isomorphic Labs has been established under the London-based AI lab DeepMind, which is also a subsidiary of Alphabet. DeepMind has already experienced success in AI-enabled drug discovery as in 2020 it released AlphaFold, an AI model for predicting protein structures.
Over the last two years, the company has made over 90 investments in the AI-enabled drug discovery space that are designed to act as a complement to existing activities in Google’s pharma-focused ventures such as Verily Life Sciences, Calico Life Sciences, and the aforementioned Isomorphic Labs.
Verily alone has raised over $1 billion to pursue its mission of enabling precision or personalized medicine which is a medical model that separates people into distinct groups with medical decisions, practices, interventions and/or products being customized to the individual patient based upon their predicted response or risk of disease.
Google’s investments in precision medicine are varied including companies that combine research, clinical and non-clinical data to customize drugs and healthcare to each patient’s specific needs. However, the company has eight important strategic priorities with regards to the application of AI: drug R&D technology, gene therapy, genomics, reproductive health, healthcare supply chains, AI radiology, backend operations automation and medical data repositories. It is in these areas that its investment, acquisition and partnership efforts are primarily focused.
CONCLUSION
The great lesson of artificial intelligence is the enduring power of human intelligence. Innovation in this space will not be determined by the brute force operational efficiencies of AI but rather by the non-linear thinking, capacity for collaboration and leadership of human beings. Those inherently scarce intangibles will determine which organizations thrive and which will be constrained by poor internal culture and inadequate leadership.
Venture capital investors must remain cognizant of this as they attempt to facilitate the growth of the industry through financing and related support. The potential for AI to enhance efficiency, reduce costs and improve the overall quality and quantity of drugs discovered must be tempered by the reality that there are no shortcuts in science.
It is going to take time and a great degree of iteration to truly understand what AI can and cannot do for drug discovery. Success in the application of AI to the development of the drugs that we put in our bodies will require visionary commitment on the part of senior leadership and the ability to win the enthusiastic support of scientists and workers who may not initially understand the implications of AI to their particular job function.
It is in times of such uncertainty that true leadership is required, and the ongoing AI evolution will be no different. Yet, it is precisely this kind of leadership that the true innovators in the entrepreneurial finance community as well positioned to assist in the development of.
Interested in the full research paper?
Join to receive Venture Capital research, guides, models, career tips, and many other great insights delivered straight to your inbox.